PREV
PREDICTIVE ANALYTICS
NEXT
Analyzing and predicting time series with statistical models and machine learning to support strategic decision-making processes
Predicting time series in aHead Research
The primary focus of our specialists in aHead Research is to analyze and understand the client’s business priorities and needs, and then to identify the most adequate kind of prediction that can support a profitable decision-making process. The areas that may benefit from such analysis of time series are, among others: demand planning, workforce optimization, preditive maintenance and assortment planning.
The prediction of time series involves a vast and heterogeneous array of applications, including the forecast of demand, economy, production, finances, environmental variables, trends and macro-trends, diagnostics data and so forth.
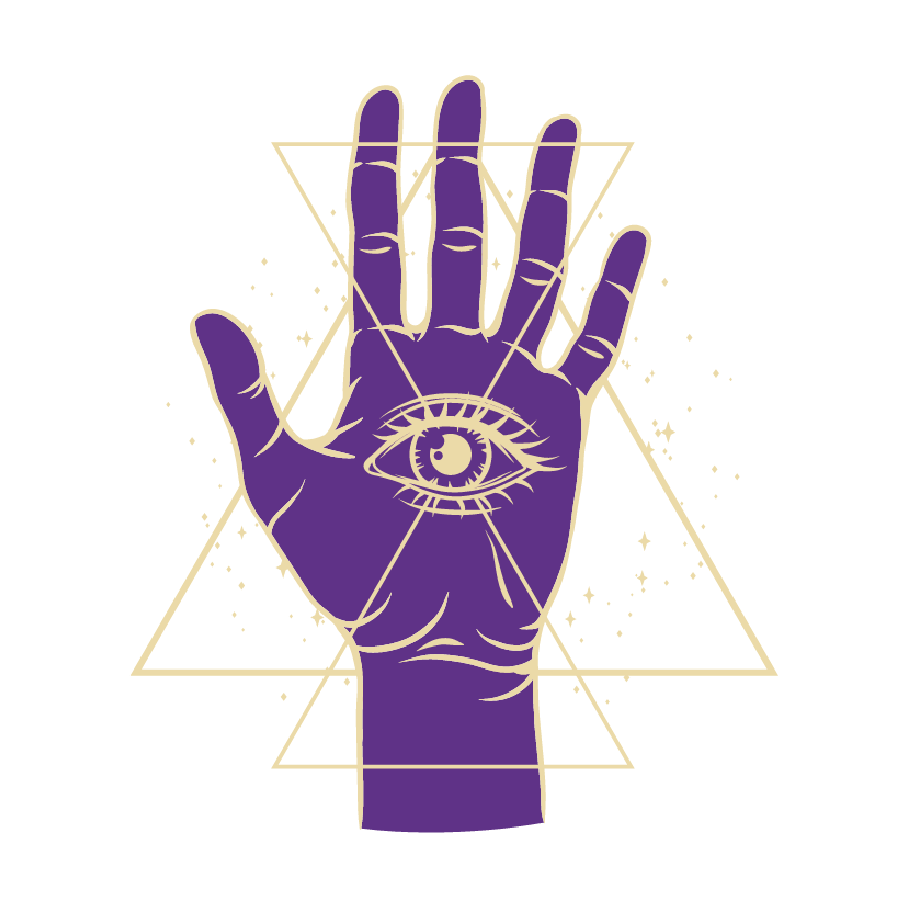
Predictive support
Predicting time series is not an infallible process, nor is it applicable to every situation. The goal of the specialists in aHead Research is to provide an analysis model able to produce a set of rules to understand in which circumstances prediction is profitable. Indeed, the analysts lead the integration of a certain predictive strategy by identifying both its benefits and limitations in the decision-making process. Our specialists are well aware of the margin of error of prediction, and they tackle it by applying a risk-based approach. Our predictive strategies involve automated tools for monitoring the predictive accuracy, the quality of the input data and the eventual presence of random fluctuations that may indicate outlier/novelties.
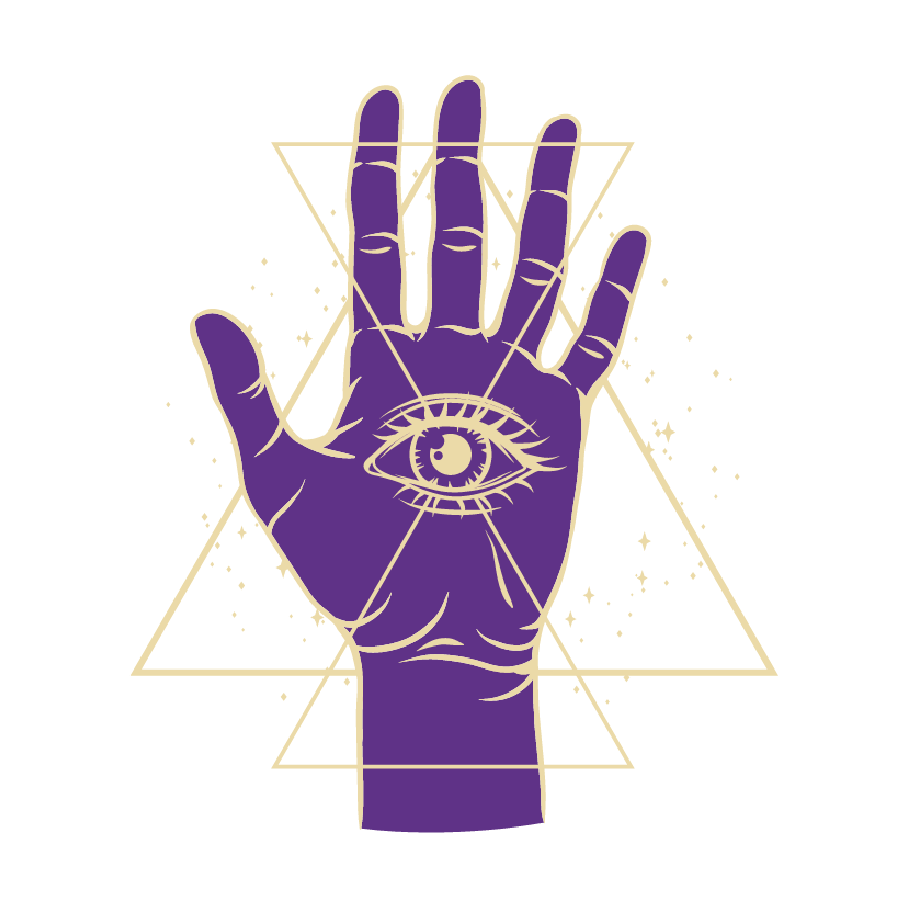
Predictive support
Predicting time series is not an infallible process, nor is it applicable to every situation. The goal of the specialists in aHead Research is to provide an analysis model able to produce a set of rules to understand in which circumstances prediction is profitable. Indeed, the analysts lead the integration of a certain predictive strategy by identifying both its benefits and limitations in the decision-making process. Our specialists are well aware of the margin of error of prediction, and they tackle it by applying a risk-based approach. Our predictive strategies involve automated tools for monitoring the predictive accuracy, the quality of the input data and the eventual presence of random fluctuations that may indicate outlier/novelties.
Predictive models
In aHead Research, we are devoted to transferring technologies from the research domain to the industrial areas. Therefore, our goal is to provide our clients with cutting-edge statistical and machine learning models within the realm of analysis and forecast of historical series. The analysis of time series requires the development of models able to interpret the underlying causes of certain data odserved. Regarding the predictive models based on machine learning, oour predictive stack employs neural network tehnologies -RNN, GRU, LSTM- and autoregressive statistical models. The former are specifically suitable for historical series that have complex trends, with correlations between moments over long periods of time, possible deficiencies in data and that require long-term forecasting. The latter, on the hand, are autoregressive models -VAR, Bayesian VAR, SARIMA, ARIMAX- and are capable of predicting series with strong seasonal components, as well as long-term trends. Among the statistical models, we also employ cutting-edge algorithms such as GAM (Generalized Additive model), which allow for the interpretation of a certain prediction through the individual components of an historical series (trends, seasonal developments, etc.). Scientific research in collaboration with our university partners has made it possible to develop predictive models for historical series based on the Transformers, which have high potential in terms of predictive accuracy and efficiency and may optimize many commonly used algorithms.
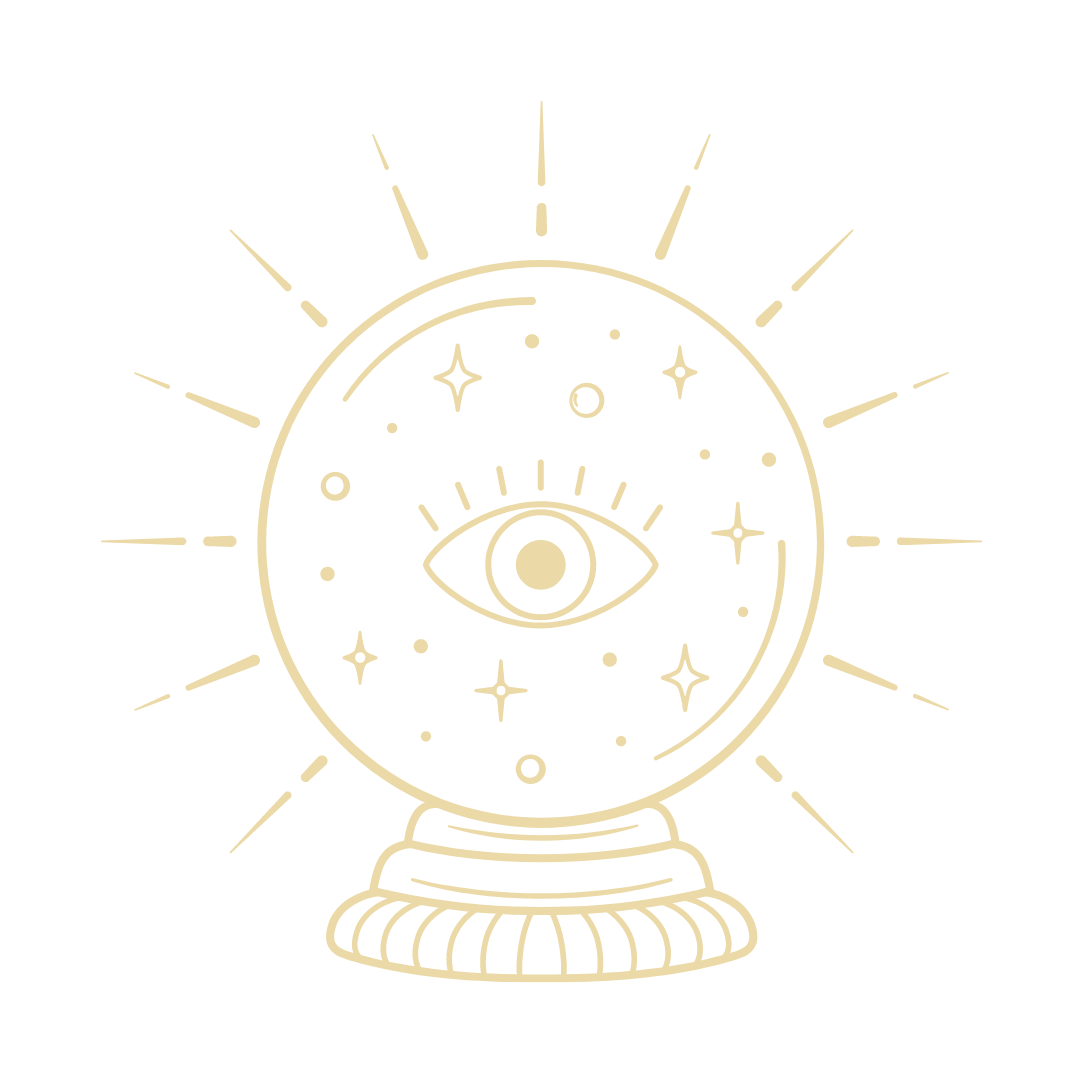
Predictive models
In aHead Research, we are devoted to transferring technologies from the research domain to the industrial areas. Therefore, our goal is to provide our clients with cutting-edge statistical and machine learning models within the realm of analysis and forecast of historical series. The analysis of time series requires the development of models able to interpret the underlying causes of certain data odserved. Regarding the predictive models based on machine learning, oour predictive stack employs neural network tehnologies -RNN, GRU, LSTM- and autoregressive statistical models. The former are specifically suitable for historical series that have complex trends, with correlations between moments over long periods of time, possible deficiencies in data and that require long-term forecasting. The latter, on the hand, are autoregressive models -VAR, Bayesian VAR, SARIMA, ARIMAX- and are capable of predicting series with strong seasonal components, as well as long-term trends. Among the statistical models, we also employ cutting-edge algorithms such as GAM (Generalized Additive model), which allow for the interpretation of a certain prediction through the individual components of an historical series (trends, seasonal developments, etc.). Scientific research in collaboration with our university partners has made it possible to develop predictive models for historical series based on the Transformers, which have high potential in terms of predictive accuracy and efficiency and may optimize many commonly used algorithms.
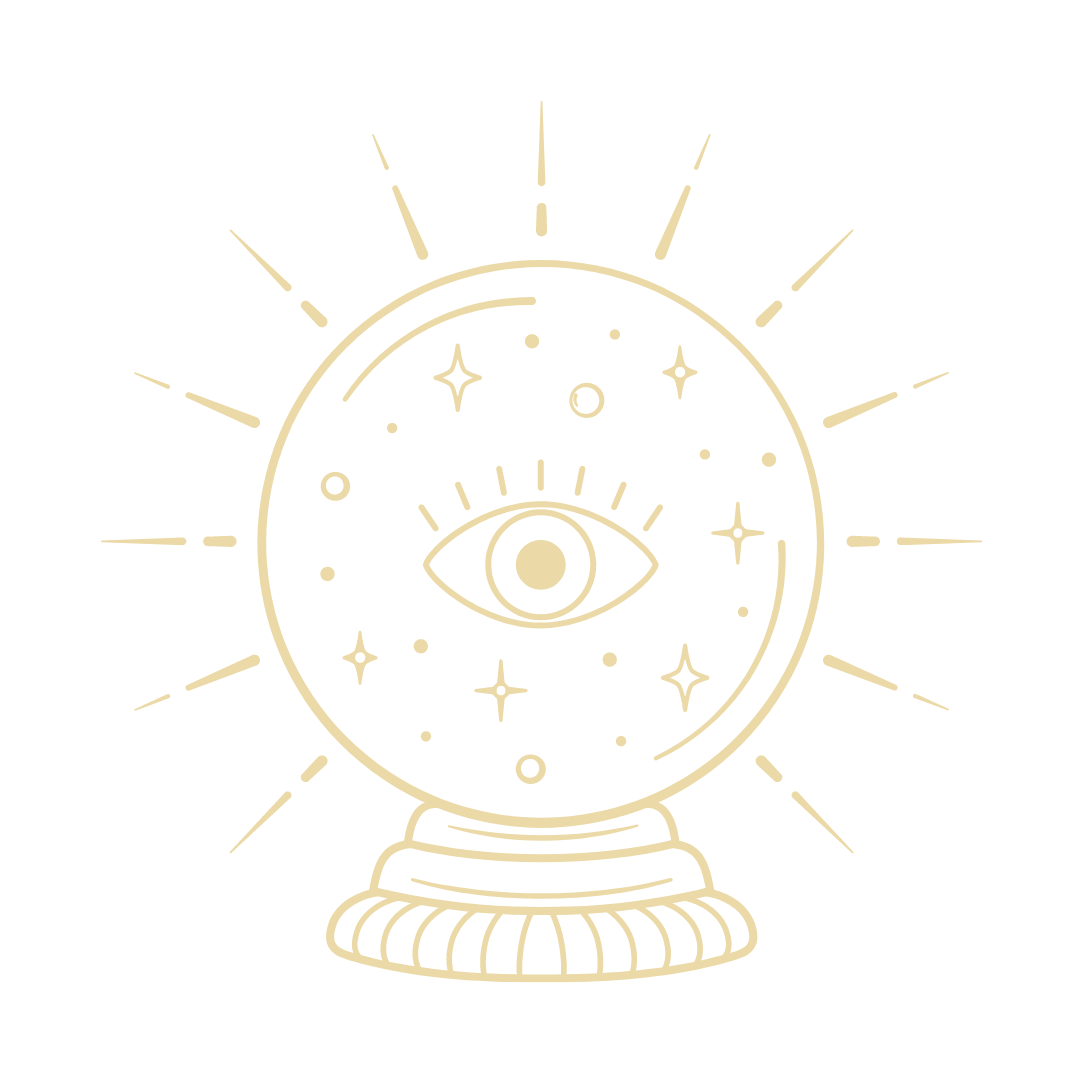
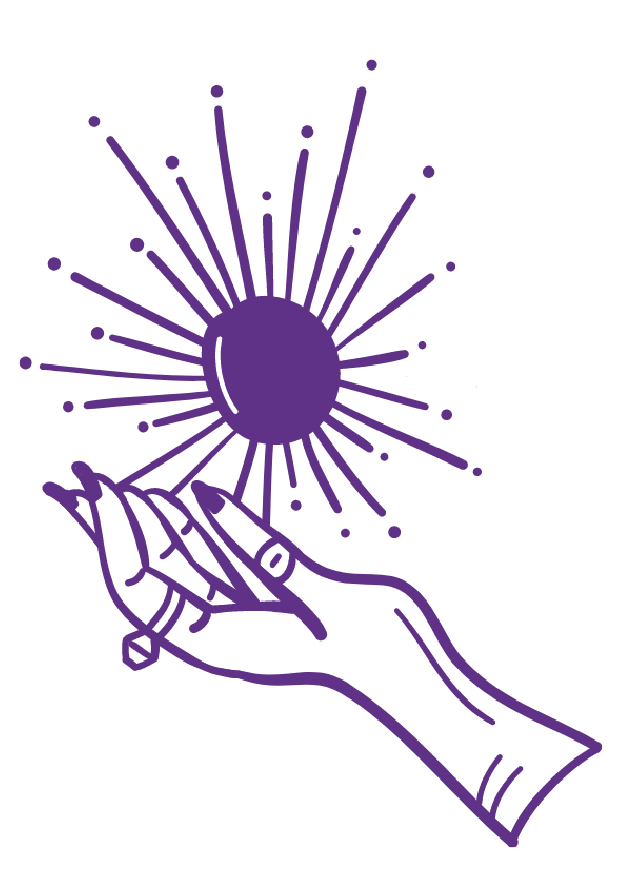
Machine learning for the prediction of time series
Automated learning is what allows a system to learn authonomously and implement its performances solely through data, without the need for custom programming. It is an extremely powerful tool that should be employed in any situation where data can anticipate or explain changes in the progression of the historical series. When a large amount of data is fed to a machine learning system, such system can identify relationships that the human eye is not able to perceive, and therefore better decisions. For instance, in predicting demand, machine learning may support the automated interpretation of variations caused by seasonality and price changes, sales of other events.
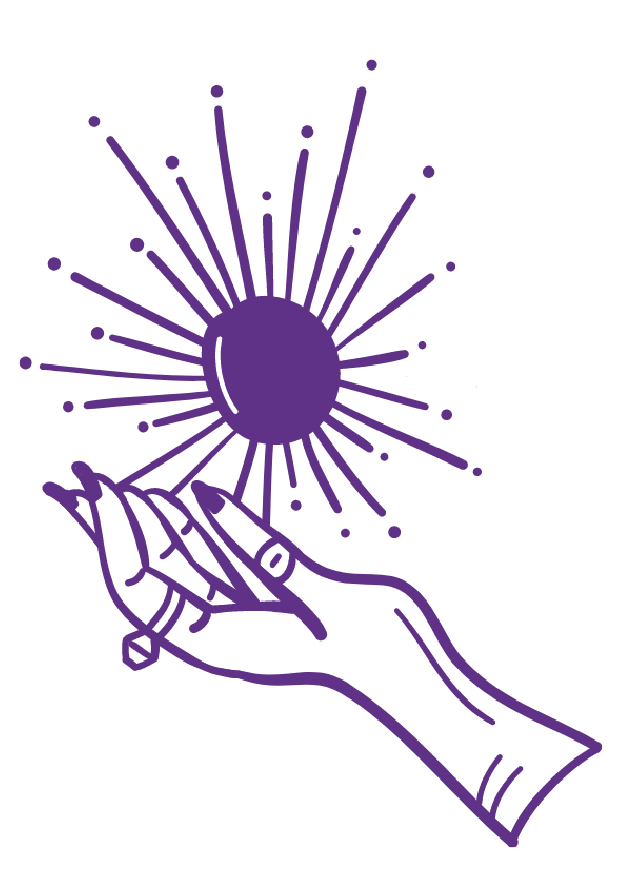
Machine learning for the prediction of time series
Automated learning is what allows a system to learn authonomously and implement its performances solely through data, without the need for custom programming. It is an extremely powerful tool that should be employed in any situation where data can anticipate or explain changes in the progression of the historical series. When a large amount of data is fed to a machine learning system, such system can identify relationships that the human eye is not able to perceive, and therefore better decisions. For instance, in predicting demand, machine learning may support the automated interpretation of variations caused by seasonality and price changes, sales of other events.
Predictive strategies
Our predictive strategies are based on a methodological framework that, through reusable software components, allows for: ensemble prediction -where the prediction is the result of the elaboration of multiple models-, iper-parameters optimization -in which the optimization strategies are the result of the collaboration with the team of Operative Research in aHead Research- and AutoML techniques for the optimized and automated management of thetraining and evaluation of the models -e.g. MLOps.
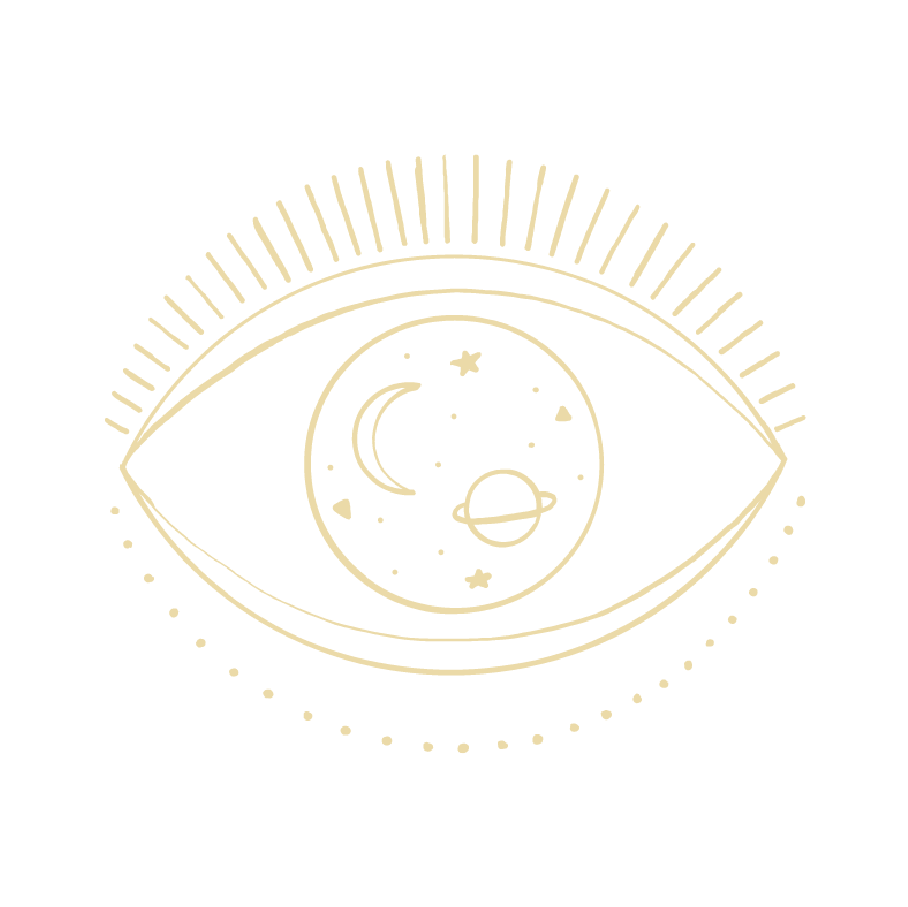
Predictive strategies
Our predictive strategies are based on a methodological framework that, through reusable software components, allows for: ensemble prediction -where the prediction is the result of the elaboration of multiple models-, iper-parameters optimization -in which the optimization strategies are the result of the collaboration with the team of Operative Research in aHead Research- and AutoML techniques for the optimized and automated management of thetraining and evaluation of the models -e.g. MLOps.
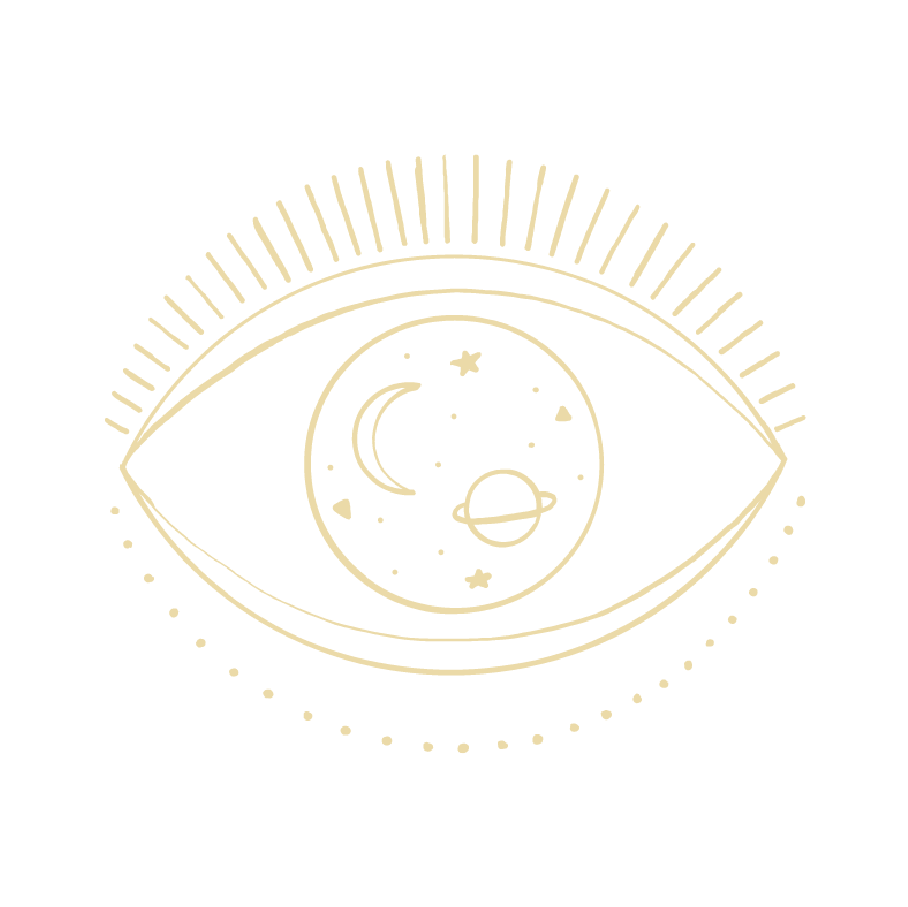
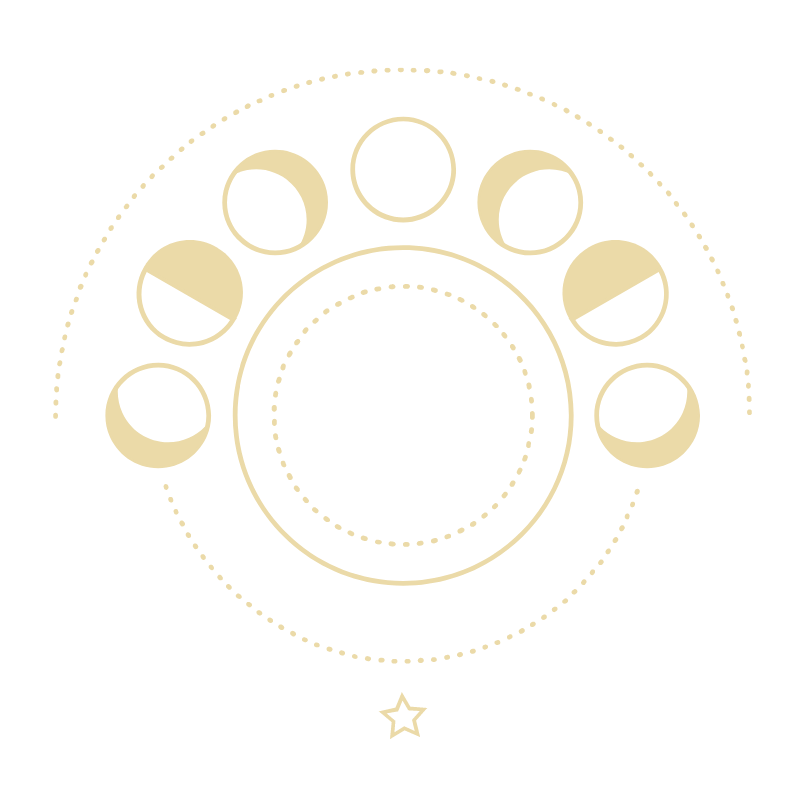
Analysis of the historical series
Our goal is not only to provide the prediction of a given historical series, but also to enhance the value of the information intrinsically contained in the series, in order to turn it into value for the business. We offer strategies to break down the historical series in order to identify trends, seasonality, specific statistical events and outliers. From a given historical series, we can extract more than 70 statistically specific features, all of which can be used by machine-learning systems to support processes of classification or regression. Lastly, we employ Change-Points analysis to identify in which points the probability distribution of the stocastic processes associated with a historical series change. This kind of analysis allows, for example, for the localisation of changes in trends and for an estimate of their intensity.
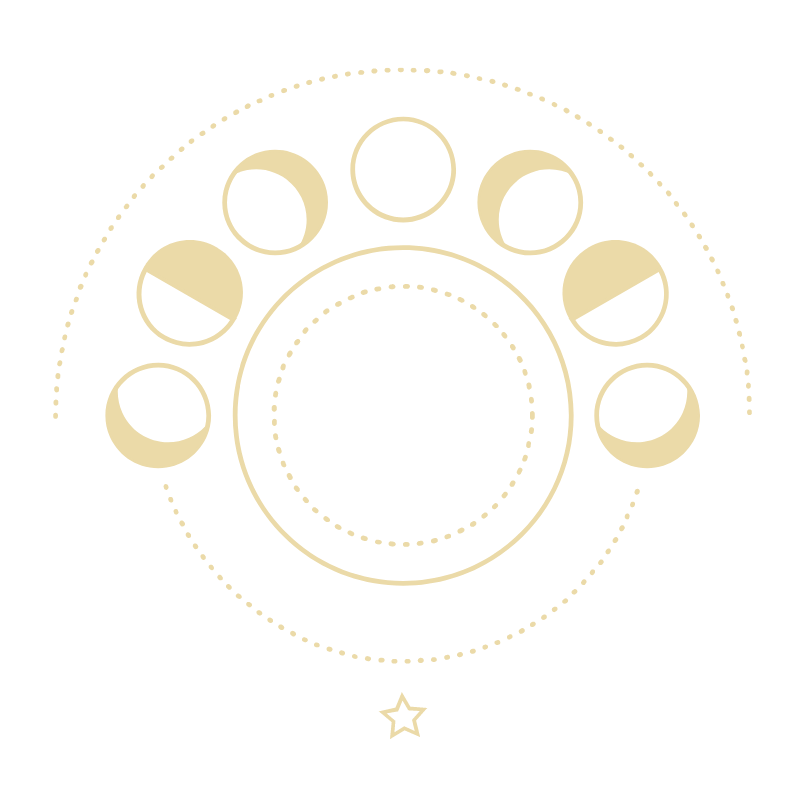
Analysis of the historical series
Our goal is not only to provide the prediction of a given historical series, but also to enhance the value of the information intrinsically contained in the series, in order to turn it into value for the business. We offer strategies to break down the historical series in order to identify trends, seasonality, specific statistical events and outliers. From a given historical series, we can extract more than 70 statistically specific features, all of which can be used by machine-learning systems to support processes of classification or regression. Lastly, we employ Change-Points analysis to identify in which points the probability distribution of the stocastic processes associated with a historical series change. This kind of analysis allows, for example, for the localisation of changes in trends and for an estimate of their intensity.